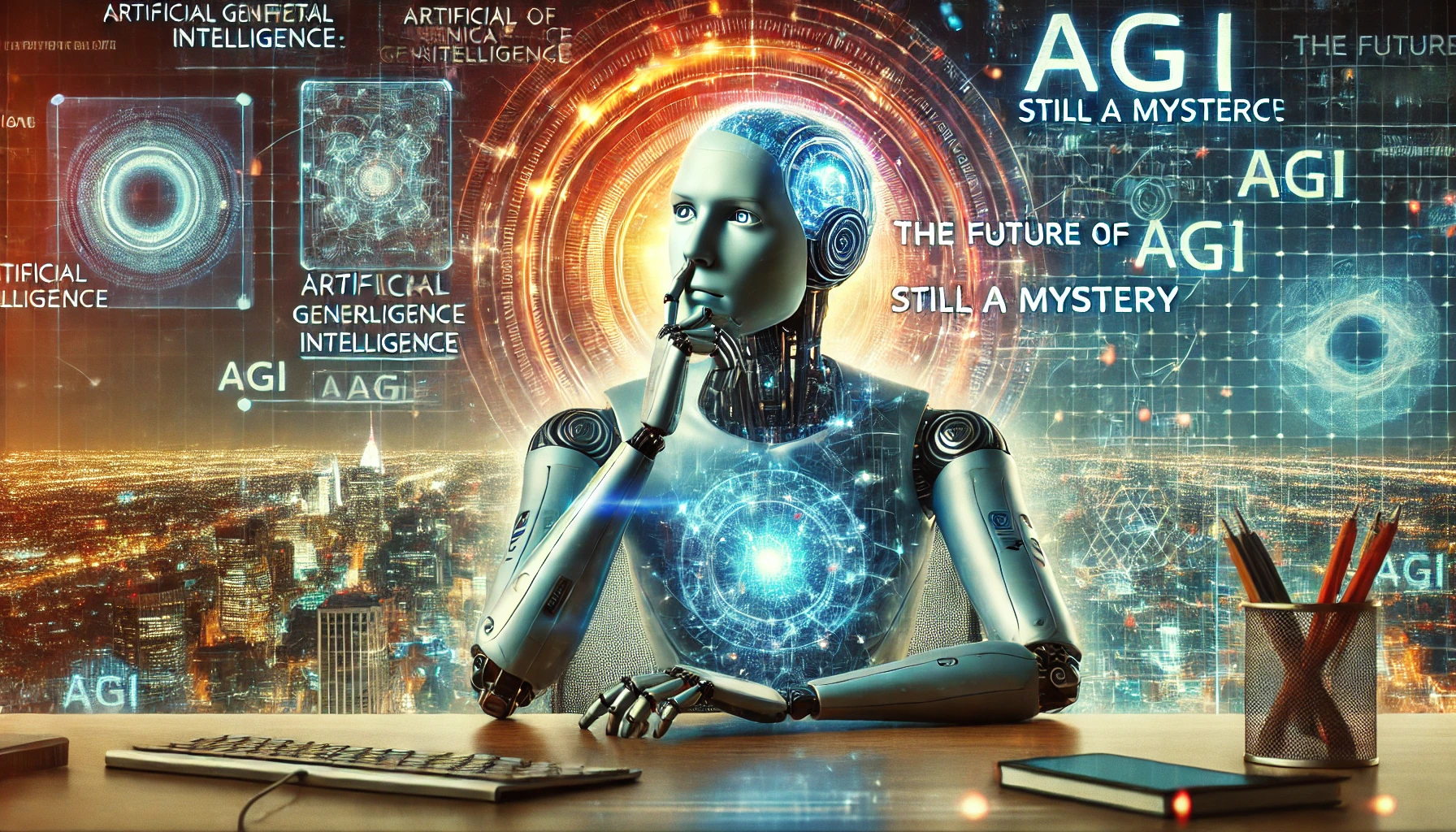
Artificial General Intelligence (AGI) has become one of the most debated and elusive concepts in the field of artificial intelligence. While there have been tremendous advancements in AI, especially in narrow domains like natural language processing and image recognition, AGI represents a much more complex challenge. It aims to replicate human-like intelligence across a broad spectrum of tasks, transcending specific areas of expertise. Yet, despite the excitement surrounding AGI, even the world’s top AI researchers, including Fei-Fei Li, widely known as the “godmother of AI,” admit to not fully understanding what AGI truly entails.
What is AGI?
In simple terms, AGI refers to AI systems that can perform any intellectual task that a human being can do. Unlike current AI systems, which excel at specific tasks (also known as narrow AI), AGI would be capable of learning and reasoning across various domains. However, one of the core issues is that there’s no universally accepted definition of AGI. OpenAI, a leader in AI development, has been attempting to create a framework by categorizing AI into five progressive stages: from basic chatbots, like ChatGPT, to more advanced entities that can reason, innovate, and potentially operate like entire organizations.
Sam Altman, CEO of OpenAI, has described AGI as akin to a “median human” who could be hired as a coworker. This analogy, however, has been met with skepticism, as some argue that this level of intelligence goes far beyond what we consider human capability, especially when considering how AI might innovate or manage entire organizations.
Fei-Fei Li’s Thoughts on AGI
Fei-Fei Li, a leading figure in AI and responsible for groundbreaking work in computer vision and machine learning, has openly admitted her confusion regarding AGI. Speaking at the Credo AI Responsible Leadership Summit, Li explained that, despite her extensive expertise, she does not know what AGI truly is or whether it will ever be achieved. Her admission highlights the complexity and ambiguity surrounding AGI, even among those who have been instrumental in shaping the current AI landscape.
Li’s career has been marked by significant contributions to AI, notably her work on ImageNet, a dataset that revolutionized AI research in the early 2010s. Yet, when it comes to AGI, even she finds it hard to grasp what a true general intelligence might look like or how we would measure its achievement.
This uncertainty is not unique to Li. Many researchers in the field echo similar sentiments. AI pioneer Sara Hooker, for instance, has argued that questions about AGI are less about technical achievements and more about philosophical inquiries—such as what it means for a machine to truly “understand” or “think” like a human.
Why is AGI So Hard to Define?
The challenge of defining AGI lies in its scope and ambition. Current AI systems excel at specific tasks for which they have been explicitly trained, such as playing chess, analyzing medical images, or generating human-like text responses. However, these systems do not possess the ability to transfer knowledge between domains or demonstrate an understanding of the world akin to human cognition.
AGI, on the other hand, would need to do much more. It would require not only problem-solving skills but also the ability to learn new tasks independently, adapt to unforeseen circumstances, and make decisions in environments that are vastly different from its training data.
One of the biggest challenges in achieving AGI is “transfer learning”—the ability of a system to apply knowledge gained in one context to a completely different scenario. Humans do this effortlessly; for example, you might use knowledge from cooking to help understand chemistry or physics. AGI, in contrast, would need to replicate this form of adaptive, cross-domain reasoning, which is currently far beyond the capabilities of even the most advanced AI systems.
The Skepticism Surrounding AGI
Many AI experts remain skeptical about whether AGI can ever be realized. Some argue that the concept of AGI may be fundamentally flawed, as it presupposes that human-like intelligence can be replicated in machines. Others question the practicality and ethics of pursuing AGI. For instance, if AGI were to be developed, it could potentially surpass human intelligence, raising questions about control, safety, and societal impact.
A significant part of the skepticism also comes from the lack of a clear benchmark for AGI. Unlike narrow AI, where success can be measured through specific tasks (e.g., AI beating a human in a game of chess), it’s unclear how we would evaluate whether a machine has achieved AGI. Some have proposed tests, such as Steve Wozniak’s “coffee test,” which asks whether a machine could enter a random house and make a cup of coffee, interacting with its environment like a human would(Columbia Engineering). But even this test, while illustrative, does not capture the full breadth of what AGI would need to do.
The Ethical Dilemmas of AGI
Even if AGI were achievable, its development would be fraught with ethical challenges. Fei-Fei Li herself has been an advocate for responsible AI development and regulation. She emphasizes that the goal of AI research should not just be technological advancement but also understanding the societal impacts that such advancements could bring.
Li’s involvement in policy discussions, particularly around AI regulation in California, demonstrates her commitment to ensuring that AI, including any future AGI developments, is developed with human safety and well-being in mind. She argues that the focus should be on minimizing harm and maximizing benefits to society, rather than punishing developers when things go wrong, as was the case with California’s SB 1047, a bill aimed at regulating AI.
What’s Next for AGI?
Despite the uncertainty surrounding AGI, research in the field continues. Companies like OpenAI, Google DeepMind, and others are investing heavily in developing systems that inch closer to general intelligence. But according to Fei-Fei Li, achieving AGI is likely still far in the future. In her view, understanding the complexities of human intelligence, particularly spatial and perceptual intelligence, may be one of the biggest hurdles AI researchers face(WireFan).
Rather than focusing solely on language models, which have garnered the most attention in recent years, Li believes the next frontier in AI is about bridging the gap between “seeing” and “doing.” This will involve building systems capable of understanding and interacting with the world in a way that goes beyond mere pattern recognition.
Conclusion
AGI remains one of the most intriguing yet controversial topics in AI research. While narrow AI systems have made remarkable progress, the development of a truly general intelligence capable of performing any intellectual task remains elusive. As Fei-Fei Li and other top AI researchers have pointed out, AGI’s very definition is still up for debate, and whether it can be achieved remains an open question.
As the field of AI continues to evolve, the conversation around AGI will likely shift from one of pure technological ambition to one that includes a broader understanding of ethics, societal impact, and the true nature of intelligence itself.